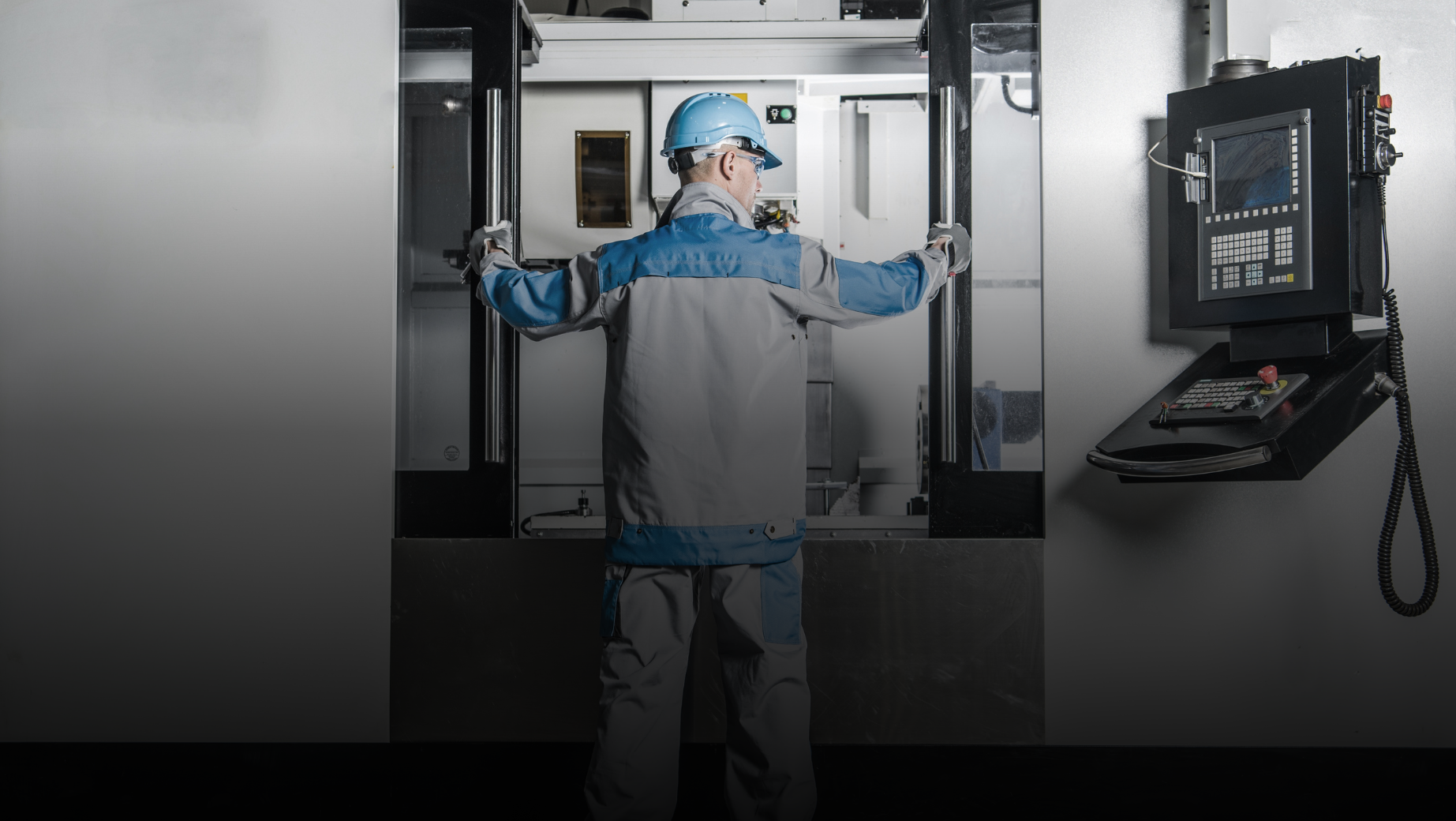
Understanding Predictive Maintenance: Technology, Challenges, and Opportunities
|12 February 2024In the world of complex industrial machines, effective planning for operation and maintenance is important. If a machine breaks down unexpectedly, it can slow down the whole production process. To mitigate this, manufacturers often rely on machines with a solid maintenance history, efficient repair teams, and backup machines. However, these measures can be costly and don’t always prevent downtime.
To improve this situation, efforts have been made to improve maintenance planning, such as detailed logging of machine failures and monitoring of relevant parameters. But what would really help is a system that can predict failures before they occur. Imagine if we could know when a machine needs maintenance before it breaks down. This is what Predictive Maintenance (PdM) can do.
This article will explain how PdM can save costs, improve efficiency, and reduce downtime. It uses advanced analysis and data to predict when equipment might fail. We’ll look at how it works, from detecting faults early to examples of its use in the real world.
This article will discuss how PdM, through advanced analytics and data-driven insights, can predict early equipment degradation and potential equipment failures. We’ll delve into its mechanisms, from the science behind early fault detection to real-world applications.
We’ll also examine the challenges, engage with experts, and look at future trends. By the end, you’ll have a comprehensive understanding of predictive maintenance, a technology that’s set to change the way we think about maintenance and operations. Let’s get started!
What is Predictive Maintenance?
PdM is a proactive approach that involves regular monitoring of equipment to assess its condition, predict future trends, and plan maintenance activities in advance. It encompasses equipment condition monitoring, fault diagnosis, residual life prediction, and maintenance decision-making. The goal is to provide timely and relevant information to the maintenance and production teams, which aids in extending maintenance intervals and reducing unplanned outages and unexpected breakdowns.
PdM is a key element of the Industry 4.0 paradigm, enhancing other maintenance and production planning methods with just-in-time work. It operates on the premise that monitored machine parts undergo measurable degradation processes, enabling the estimation of time windows for maintenance activities. The benefits of PdM include optimized part usage, reduced costs, extended machine lifespan, improved plant safety, enhanced product quality with near-zero manufacturing failures, fewer accidents, and easy integration with organizational planning.
The PdM system architecture comprises data acquisition, analysis, condition monitoring, health evaluation, prognostics, decision support, and a user interface. It utilizes various data acquisition techniques, such as acoustic and thermal imaging, to identify vulnerabilities and boost operational efficiency. In a nutshell, PdM is about using data to anticipate and plan for maintenance, thereby improving efficiency and reducing downtime.
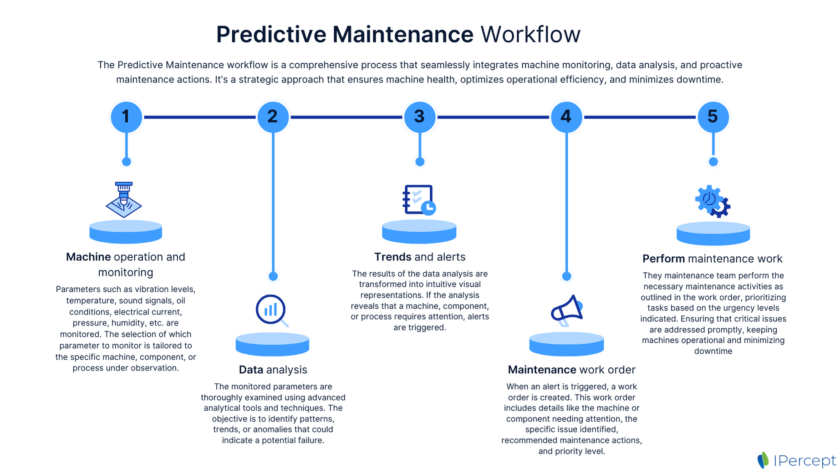
Maintenance Strategies in Industrial Environments
In the industrial landscape, various maintenance strategies are employed to ensure the smooth operation of machinery. These strategies, each with their own benefits and challenges, play a pivotal role in the overall productivity of a manufacturing unit.
Corrective Maintenance (CrM) is a reactive approach where maintenance actions are postponed until a failure occurs. This strategy accepts the risk of asset unavailability, which can lead to unexpected downtime and potential production losses.
Preventive Maintenance (PvM) is a proactive approach that schedules maintenance interventions in advance. This results in the replacement of spare parts while they are still functional, effectively reducing their lifespan. Although this approach can prevent sudden failures, it can also lead to unnecessary maintenance activities and increased costs.
The ideal maintenance strategy should aim to improve the condition of equipment, decrease failure rates, and minimize maintenance costs, while maximizing the lifespan of the equipment. This is where Predictive Maintenance (PdM), the focus of our discussion, comes into play.
PdM is a strategy where maintenance is performed based on an estimate of the health status of a piece of equipment. This approach aims to maximize the time of use and operation of equipment, delay or reduce maintenance activities, and reduce material and labor costs. By predicting when a component of the machine is likely to fail, PdM allows for timely intervention, preventing unexpected breakdowns and minimizing downtime.
At the heart of PdM is the continuous monitoring of critical components and subsystems of complex industrial machines. This is achieved through the use of advanced sensors and data analysis tools that provide insights into the health status of these components. By continuously tracking parameters such as vibration, temperature, and pressure, maintenance teams can gain a comprehensive understanding of the operational health of the equipment. This continuous monitoring not only enables the prediction of potential failures but also provides maintenance teams with the opportunity to intervene proactively, thereby preventing costly downtime and extending the lifespan of the equipment.
However, implementing PdM is not without its challenges. It requires sophisticated data recording and analysis capabilities, and a deep understanding of the equipment’s operation. Despite these challenges, the opportunities presented by PdM are significant.
Harnessing Predictive Maintenance – a conversation with Jan Erickson
Jan Erickson is our seasoned Senior Advisor at IPercept, with a deep-rooted expertise in Condition Based Maintenance. His impressive career spans over two decades at Volvo GTO and 15 years in process industry working with PdM/CBM. Jan’s principle: “Make your decision based on facts”, demonstrates his fact-driven approach to maintenance. His vast experience and practical knowledge make him a key part of our team.
Jan Erickson spoke to us about the role of PdM, harnessing PdM for critical machines and processes, and understanding the Return on Investment (ROI) of PdM.
What are the essential components of a robust PdM system?
Jan Erickson: A robust PdM system is built on a solid maintenance strategy and a clear vision. This is in line with our view that a well-defined strategy is the foundation of any successful manufacturing and upkeep process. It provides direction and helps to align the organization’s efforts toward a common objective. A PdM system should also support secure data management, process management, bill-of-materials visibility, and other features that can simplify maintenance planning.
What skills are necessary for a team to effectively implement and manage a PdM system?
Jan Erickson: Machine-specific knowledge and an understanding of each machine’s tolerances are crucial skills for a team implementing PdM. The emphasis should be on technical expertise at multiple levels and domains, including machine domain knowledge and data interpretation. Continuous learning and upskilling are key in keeping up with the changing demands of smart manufacturing and maintenance.
How do you measure the effectiveness of a PdM system?
Jan Erickson: It’s important to know the tolerances of both the machine itself and the products it produces. Maintenance costs, productivity, overall equipment effectiveness (OEE), and tool replacements can all be quantified to determine the effectiveness of a PdM solution. A maintenance solution should be evaluated using tangible metrics. There should also be a focus on organizations developing clear key performance indicators (KPIs) for their PdM initiatives.
How does predictive maintenance change the dynamics for production and maintenance teams?
Jan Erickson: Predictive Maintenance (PdM), or Condition Based Monitoring (CBM), allows for planned production time. This is made possible through fact-based decision support systems, which make production and maintenance more cost-effective.
What are the primary indicators that a production line might be a suitable candidate for predictive maintenance?
Jan Erickson: The suitability for PdM largely depends on the management’s approach to machine operation. If the production management is comfortable running the machines until they fail, then there is no need for PdM or CBM. However, if this approach is not acceptable, then PdM should be implemented. It’s all about the organization’s tolerance for risk and downtime.
Navigating the challenges
Developing Competency: PdM presents a unique set of challenges that require careful navigation. One of the key hurdles is the development of machine-specific knowledge. Understanding the nuances of each machine and manufacturing processes is crucial for providing accurate and actionable maintenance insights. This requires a careful integration of mechanical engineers and data scientists, who possess a deep domain understanding of the unique mechanical attributes of machines.
Data Quality and Structure: Other challenges include technical aspects such as the quality and structure of data recorded or captured from machines and manufacturing processes. These factors influence the granularity of insights and the effectiveness of PdM strategies.
No one-size-fits-all solutions: Every machine is unique and requires highly customized solutions. Configuring PdM solutions to the specifics of each machine and its subsystems can be challenging. However, the way forward lies in solutions that have self-configuring algorithm capabilities for all key mechanical subsystems, and therefore, for all machines. The key to successful customization is the use of specifically configured machine learning algorithms. These algorithms leverage domain knowledge on the machines and processes, enabling effective predictive maintenance strategies.
Use IPercept’s solution to run your predictive maintenance programs
At IPercept we provide AI-based, hardware-enabled software solutions and services that can best be described as the fitness tracker for complex industrial machines. At the push of a button, you can learn about a machines’ health, down to micrometer changes for each critical component. You can get insights into what maintenance activities you should apply and when. You can monitor how the machines are operated, how to optimize their utilization and much more.
All of this is achieved through a plug-n-play installation – for old machines as well as new. All securely provided without requiring access to any machine controllers or IT-systems. These services reduce maintenance costs, downtime and increase productivity – a solid ROI for our customers.
Stay connected with us on LinkedIn and explore our website for similar content and updates from IPercept. We’re just a message away if you have any queries.