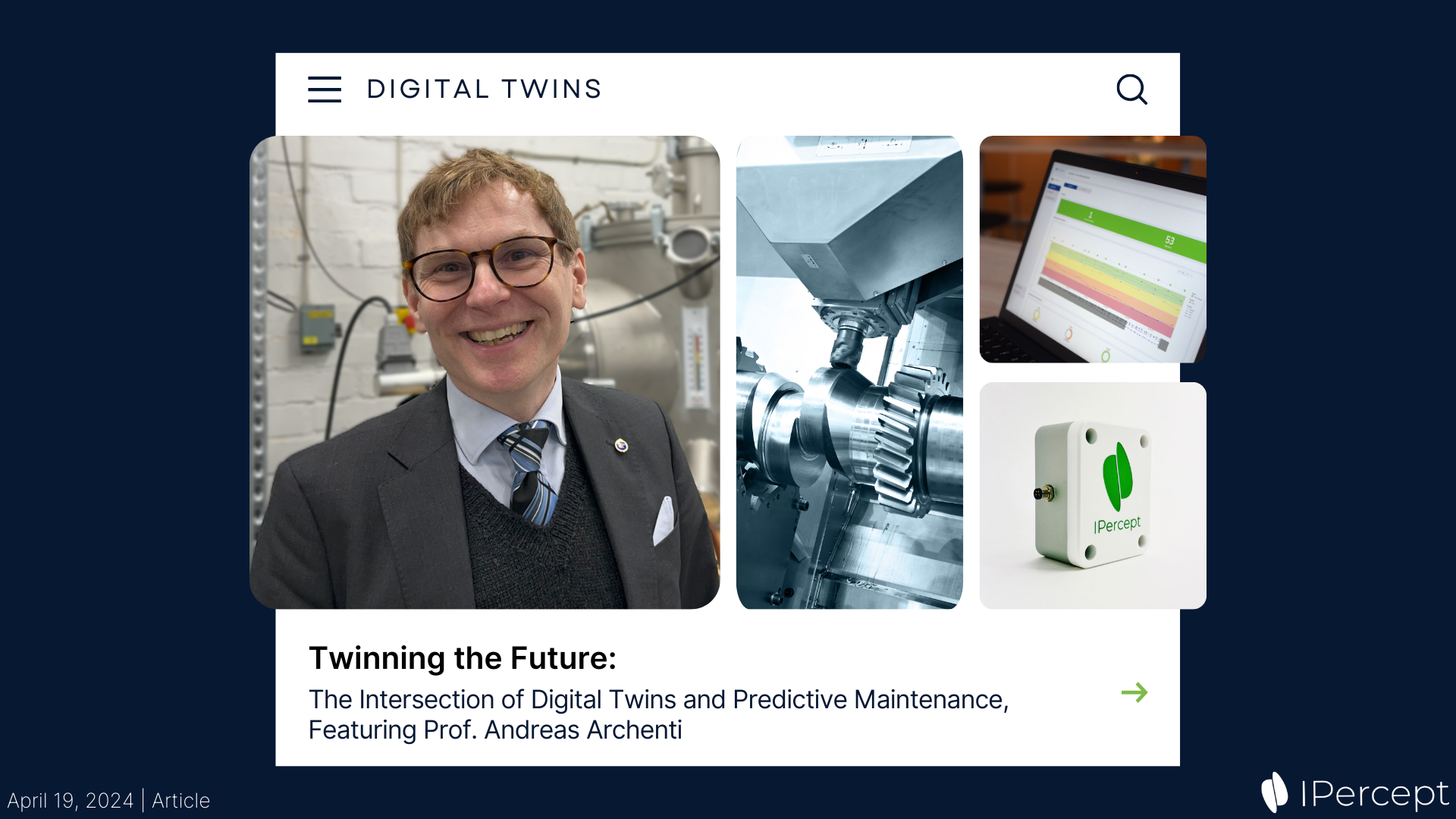
Twinning the Future: The Intersection of Digital Twins and Predictive Maintenance
|19 April 2024Industrial machinery, such as machine tools, are designed to operate for extended periods in demanding environments. In the course of operation, machines inevitably experience a degradation in health and performance. If such degradation is overlooked or not properly monitored, it can lead to unexpected machine breakdowns, disrupting the entire production process. This disruption could result in costly delays, increased maintenance needs, and a significant reduction in overall efficiency. Manufacturers have long grappled with this issue. To mitigate it, they rely on scheduled maintenance activities, machines with a solid maintenance history, efficient repair teams, and backup machines.
Efforts have been made to improve production and maintenance planning, such as detailed logging of machine failures and monitoring of relevant parameters from the machine controller. However, keeping track of these measures is a major challenge; they can be inaccurate, expensive, and do not always prevent unexpected failures. What is truly needed is the ability to track and compare the degradation of machine health at the component level, as well as performance degradation over time and across different manufacturing processes.
Imagine creating a virtual model of the machine that accurately reflects its physical counterpart. And that, this virtual model could be used to simulate various scenarios, predict outcomes, and identify failures before they occur. This exemplifies the capabilities of Digital Twins, showcasing their potential for even greater functionality.
This article will explore how Digital Twins can enable advanced machine maintenance and advanced machine operational improvement solutions. We’ll dive into how it works, and more, with insights from an expert. By the end, you’ll gain an understanding of a technology that is poised to transform our approach to industrial machine maintenance and operations. Let’s get started!
Understanding Digital Twins
Digital Twins are computerized models of a subsystem, a machine, or even an entire factory production line. At the machine level, digital twins are established using comprehensive simulations to virtually mirror a physical machine and its processes down to the component level. Consequently, they can be used to evaluate the current condition of a machine and predict future behavior. The goal is to provide timely and relevant information to the production and maintenance teams, thereby optimizing machine utilization, extending maintenance intervals, and reducing unplanned stops and unexpected breakdowns.
The advancements in advanced sensor technology and the Industrial Internet of Things (IIoT) have enhanced the accuracy of digital twins and made their integration more feasible. Models like digital twins need to be tuned with sensor or metrology data to ensure this accuracy. These advancements ensure that the digital version accurately reflects the historical data along with the current condition of the machine. Modern sensors, which are highly sensitive, accurate, and robust, enable high-quality data acquisition if applied correctly. And, IIoT systems have the capability to transfer this high-quality data to various platform, ensuring flexibility in data management and accessibility. Here, digital twin models run comparisons to historical data and ideal scenarios. They also run simulations to identify parameters that need to be adjusted to optimize machine utilization and operations. Digital twin models also have the capabilities to identify and track operational behavior that deviates from expected behavior, such as collisions in machine tools and power consumption of the machine.
Therefore, digital twins are a convergence of numerous technologies that were developed under the tide of the Industry 4.0 paradigm. Their system architecture comprises data acquisition, analysis, condition monitoring, health evaluation, process evaluation, prognostics, decision support, and a user interface. In a nutshell, a digital twin is about using data and physics to build a virtual model that mirrors the actual machine, to compare, anticipate, and plan production and maintenance, thereby improving efficiency and reducing unexpected failures.
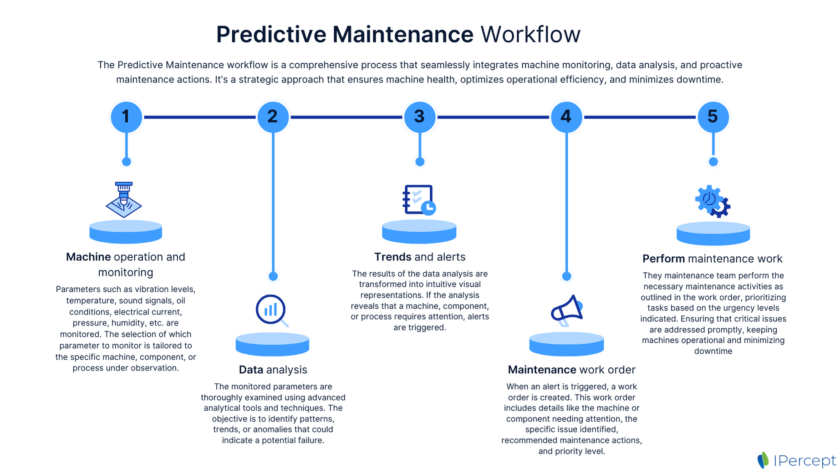
The Intersection of Digital Twins and Predictive Maintenance – a conversation with Andreas Archenti
Prof. Andreas Archenti is one of the founders and the Chief Scientist at IPercept, specializing in machinery reliability and performance. His career is distinguished by his active involvement in precision-driven research. Since 2018, he has served as a Chair Professor in Industrial Dependability at KTH Royal Institute of Technology, with a particular focus on Precision Engineering, Metrology, and Analytics. His extensive experience and academic prowess make him an invaluable member of our team.
Andreas spoke to us about the intersection of Digital Twins and Predictive Maintenance, the skills and competencies required, and the use of Digital Twins in production and maintenance.
What are the key components of a robust Digital Twin system?
Andreas Archenti: Digital Twin systems rely heavily on domain knowledge and traceability to physical phenomena. This is especially important in an era where the accuracy of the data is often overlooked.
A system, such as a machine, can be decomposed into its basic components based on their respective functions. For example, the complex system of a machine tool can be categorized into translational and rotary motion, as illustrated in the subsystems: spindles, linear axes, and rotary axes.
The process of building a physical model starts with understanding the phenomena and processes that need to be incorporated into the digital twin to yield useful results. This allows for the simulation of measurements from virtually placed sensors in a simplified environment, comparison with reality, and subsequent analysis of the differences.
What skills are necessary for a team to effectively implement and manage a Digital Twin system?
Andreas Archenti: To implement and manage a Digital Twin system effectively, a team requires a profound understanding of the domain that the DT should represent. Additionally, expertise in areas such as mechatronics, mechanical and electrical engineering, and control theory are essential ingredients. The skills needed to build a digital twin simulation of a specific machine are quite different from those needed to create a solution that can generalize across various machines. This highlights the need to understand the common features and characteristics of machines.
How do you measure the accuracy of a Digital Twin system?
Andreas Archenti: The accuracy of a Digital Twin is measured by its ability to capture a large part of a phenomenon’s complexity with the simplest possible solution. Validation and Verification (V&V) are key components and should be seen as a continuous process rather than a one-time result. In the iterations of product development, the V&V process serves as a guiding principle, assisting us in identifying the next factor to incorporate into our model for the greatest impact on output quality.
Here, concepts from the fields of precision engineering and metrology can be integrated, encompassing principles such as precision design to mitigate effects such as Abbe errors, alongside considerations of uncertainty and calibration.
“A digital twin does not need to be a perfect representation of reality; it only needs to be good enough” highlights a fundamental aspect of digital twin implementation: where the level of accuracy is set by the design requirement represented by functional performance.
How does the implementation of Digital Twins change the dynamics for production and maintenance teams?
Andreas Archenti: The use of Digital Twins brings a new dynamic to production and maintenance teams. Digital Twin simulations are a crucial tool for promoting a culture of continuous improvement. Traditional data-based solutions that depend on black box models in production have had difficulty providing enough actionable insights and scalability. Digital Twins will allow us to reach a point where we can understand and interpret our data, thereby unlocking the potential of advanced algorithms, including deep learning.
What are the key benefits of Digital Twin simulations?
Andreas Archenti: Digital Twin simulations have many benefits. The speed, depth, and validity of predictions on real physical systems are significant advantages. This enables us to reliably connect measurements with actual changes in machine health or equipment operation. The resulting actionability is where the real practical impact and value creation lies.
For example, consider the difference between these two insights: ‘There is a critical problem with your machine’ versus ‘There is a critical degradation in the nut of the ballscrew of the X1 axis.’ This level of detail can prevent a full day of downtime.
Another advantage is speed. Digital Twin simulations can be used to pretrain prediction models, eliminating the need to wait for every machine in every measurement setup to experience all the different problems and failures. For example, models can be applied across a population of machines to gain deeper insights into operational conditions and potential improvements.
Furthermore, Digital Twin simulations can aid in filtering out false positive predictions, offering a significant advantage in sustaining the efficiency and effectiveness of our systems, as well as in uncertainty budgeting for decision-making in industrial operations.
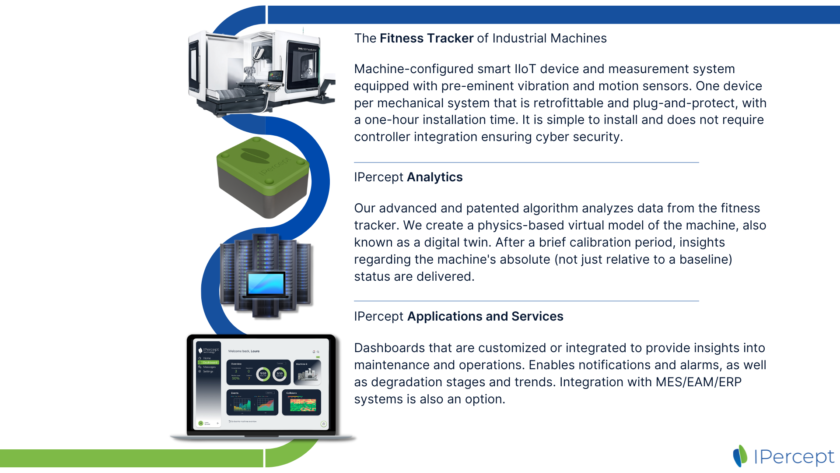
Use IPercept’s solution to run your data driven maintenance programs
At IPercept, we specialize in AI-based, hardware-enabled software solutions and services that can best be described as the fitness tracker for complex industrial machines. Our approach combines advanced sensor technology with predictive algorithms, all driven by a proprietary data stream.
This powerful combination allows you to learn about the health of your machines, detecting micrometer changes in each critical component. It provides actionable insights into necessary maintenance activities and optimal timing for them. Furthermore, it enables you to monitor machine operation, offering guidance on how to optimize their utilization and much more.
Our solutions are designed for seamless integration, offering plug-n-play installation for both old and new machines. All securely provided without requiring access to any machine controllers or IT-systems.
By reducing maintenance costs and downtime while increasing productivity, our services deliver a solid return on investment for our customers.
Stay connected with us on LinkedIn and explore our website for similar content and updates from IPercept. We’re just a message away if you have any queries.
Contact:
Christian Nordberg , Hans-Petter Andersson , Peder Venseth , or Rohan Madappa Venkatesh